Robotic Chores Made Possible
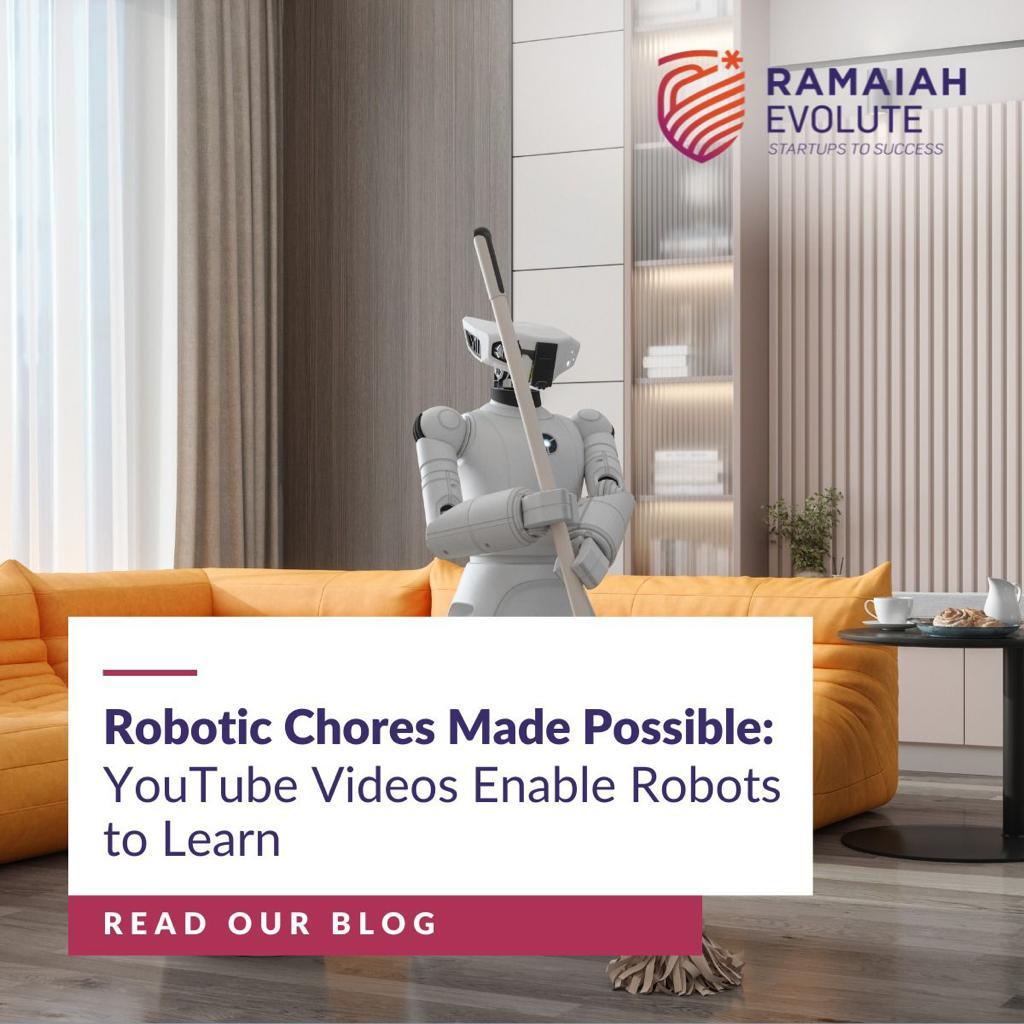
In the pursuit of true robotic learning, researchers have been exploring various solutions, and one intriguing approach involves leveraging video data. Building upon the WHIRL (in-the-Wild Human Imitating Robot Learning) algorithm developed by Carnegie Mellon University (CMU), assistant professor Deepak Pathak and his team have now introduced VRB (Vision-Robotics Bridge) as an evolution of this technology.
Similar to its predecessor, VRB trains robots by analyzing videos of humans performing tasks. However, the new system eliminates the requirement for the human demonstrations to take place in the exact environment where the robot will operate. This advancement enables the robots to perform tasks in various settings, enhancing their adaptability.
VRB focuses on extracting essential information from the videos, such as contact points and trajectory. For instance, when observing humans opening drawers, the robot learns that the contact point is the handle, and the trajectory is the direction in which the drawer opens. By analyzing multiple videos of humans opening drawers, the robot can generalize this knowledge to open any drawer effectively.
Naturally, not all drawers behave in the same way, and some may present unique challenges. To improve the robot’s performance, the researchers rely on extensive datasets for training. They leverage video databases like Epic Kitchens and Ego4D, which contains approximately 4,000 hours of egocentric videos capturing daily activities from around the world.
Shikhar Bahl, a PhD student involved in the project, highlights the vast potential of using these datasets for training robots. By tapping into the wealth of internet and YouTube videos available, this work could enable robots to learn from a wide range of sources and acquire a more comprehensive understanding of human activities.
In summary, CMU’s VRB algorithm represents a significant advancement in robotic learning. By analyzing videos of humans performing tasks, the system allows robots to adapt and explore the world more effectively. With access to extensive training datasets, including internet and YouTube videos, the potential for robots to learn from a diverse array of sources is within reach.
SHARE